What is Data Analytics? Your Complete Beginner’s Guide
Mar 1
/
Nathan Liao, CMA
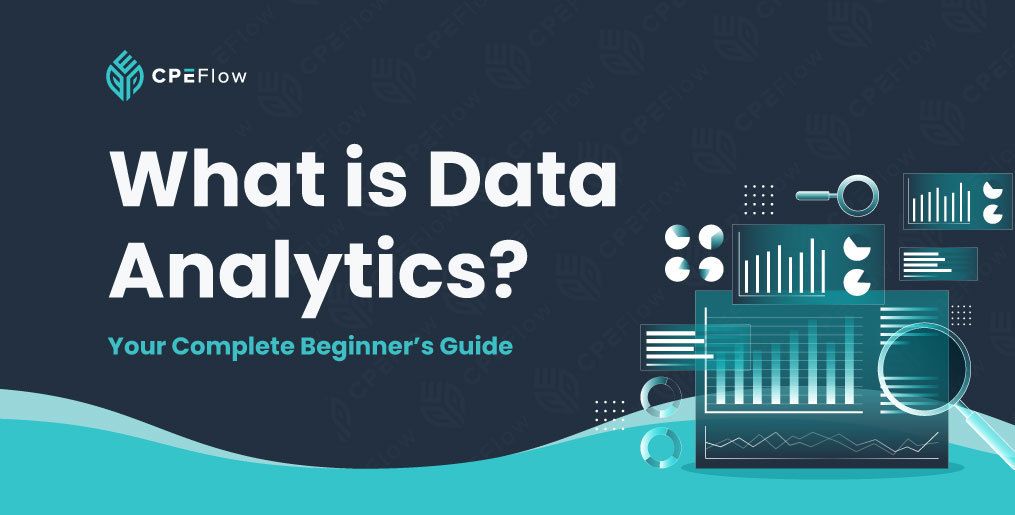
Data analytics has become indispensable in today's data-driven business landscape. Organizations increasingly rely on data analytics to drive strategic decision-making, optimize operations, and improve performance.
Understanding data analytics, from the basics to more advanced concepts, empowers accounting and finance professionals to leverage data as a strategic asset.
Below, I will cover several concepts that are crucial to understand when using data in a professional capacity.
If you want to dive deeper into this subject and earn CPE hours while you do it, look out for a presale link at the end of this blog for our engaging and enlightening Data Analytics CPE course.
Data Analytics – Key Terms to Know
Data analytics refers to techniques used to analyze raw data and extract insights to support fact-based business decisions. It involves statistical analysis, data mining, predictive modeling, and data visualization to turn complex datasets into actionable information.
To build a strong foundation in data analytics, familiarity with key terminology is essential. Some of the key terms include:
• Business Intelligence (BI): Technologies, tools, and practices for gathering, integrating, analyzing, and visualizing data to support business insights and decision-making.
• Big Data: Extremely large, complex datasets requiring advanced techniques and technologies for capture, storage, distribution, management, and analysis.
• Structured Data: Data that is organized in a standardized format and easily analyzed.
• Unstructured Data: Data without a predefined format or organization, like text, images, video, etc., requiring more advanced analytics.
• Data Mining: The process of discovering patterns and extracting knowledge from large data sets using algorithms and statistical analysis.
• SQL (Structured Query Language): Programming language used to manage and query relational databases.
• Regression Analysis: Statistical method used to estimate relationships between variables.
• Time Series Analysis: Statistical technique that analyzes data points over a period of time to forecast future trends and patterns.
• Predictive Modeling: Statistical and machine learning techniques to forecast future outcomes based on current and historical data.
• Data Visualization: Graphical representation of data insights using charts, graphs, and other techniques to convey information clearly.
Now, let’s dig into the foundations of data and gain a more robust understanding of these concepts.
Big Data and Data Analytics Foundations
You’ve probably heard the term “big data,” but do you know what it really means?
As defined above, big data refers to extremely large, complex datasets that require advanced techniques and technologies for capture, storage, distribution, management, and analysis. Big data is often differentiated by the “4 Vs.”
The 4 Vs of Big Data are:
• Volume: The enormous amount of data generated.
• Velocity: The speed at which data is generated and processed.
• Variety: Different formats and types of data.
• Veracity: Concerns regarding data quality and accuracy.
Now that we understand the foundations of big data, let’s explore different data types and how they’re used in business settings.
Get Exclusive Access & Special Discounts!
Get early bird access to new CPE courses and exclusive discounts only shared with our email subscribers.
Understanding Data Types
Data can be formatted in three different ways. Structured data is organized and easily analyzed, like the information in an Excel spreadsheet, while unstructured data, like images and videos, require more advanced analysis.
Semi-structured data is data that isn’t captured in a conventional way. This could include HTML code, graphs, or emails.
Organizing, cleaning, and analyzing structured data is simpler than semi- or unstructured data. Structured data is strictly formatted, which means data management and analysis are more efficient.
Unstructured data doesn’t have predefined attributes, making organization tricky. In most cases, unstructured data requires more complex algorithms for analysis.
From Data to Decision
The data analytics process transforms raw data, both structured and unstructured, into valuable business insights so business leaders can make data-driven decisions. Key steps in the process from data to decision include:
• Data Cleaning: Fixing inconsistencies and errors in data sets.
• Data Integration: Combining data from different sources.
• Analysis: Applying statistical models and algorithms to derive meaning.
• Interpretation: Translating analysis into strategic insights.
• Communication: Effective visualization and reporting for decision-making.
Data analysis is now a core component of many business strategies. Insights from data enable optimization, risk management, competitive advantages, and innovation.
This takes us to business intelligence.
What is Business Intelligence?
Business Intelligence (BI) leverages tools, applications, and technologies to gather, store, access, and analyze data to drive better decision-making. BI enables the creation of interactive reports, querying data, performance monitoring, predictive modeling, and data visualization.
So how do we pull and analyze data to make it useful and meaningful?
Data Mining and Analytics Techniques
Data mining is the process of extracting meaningful insights from big datasets. It involves a systematic, iterative exploration of large sets of data to uncover patterns, correlations, and trends.
Understanding the iterative nature of data mining is essential as it allows analysts to refine their models and algorithms continuously.
SQL, or Structured Query Language, is a common, standardized method of data mining.
The Role of SQL in Data Analytics
SQL (Structured Query Language) plays a fundamental role in data analytics by providing a standardized method for querying, updating, and managing databases. Its basic applications include retrieving specific data subsets, performing calculations, and filtering data based on predefined conditions.
SQL's versatility and efficiency make it a powerful tool for data analysts to extract valuable information from complex datasets.
Now, let’s move into understanding the different models used in data analytics.
Analytics Models and Types
Analytics encompasses a range of methodologies for interpreting data and making informed decisions. Four primary types of analytics include:
• Descriptive Analytics: Summarizing historical data to understand past trends.
• Diagnostic Analytics: Identifying the causes of past outcomes.
• Predictive Analytics: Forecasting future trends using statistical models.
• Prescriptive Analytics: Providing recommendations for optimal actions based on predictive insights.
Each of these analytics models provides a useful look into a business’s past, current, and future performance.
Regression Analysis and Time Series
Regression analysis is a statistical technique used to understand the relationship between one dependent variable and one or more independent variables. It helps analysts predict the value of the dependent variable based on the values of independent variables.
Time series analysis, on the other hand, focuses on analyzing data points collected over successive time intervals to identify patterns, trends, and seasonality.
Simulation and Sensitivity Analysis
Simulation models are used to replicate real-world scenarios by generating random variables based on specified probability distributions.
Sensitivity analysis examines how changes in input variables affect the output of a model.
While simulations provide valuable insights into complex systems, they are subject to limitations, such as simplifying assumptions and uncertainty in parameter estimates.
Now that we understand all the specific aspects of data, analytics, and models, it's important to know how to visualize the results and communicate them to stakeholders. After all, data and numbers don’t mean much if no one can understand the story they tell.
Visualization & Communication of Data Insights
Effective data visualization is essential for conveying complex information in a clear and compelling manner. By adhering to principles of good design, such as simplicity, clarity, and consistency, analysts can create visualizations that enhance understanding and facilitate decision-making.
Choosing the right visualization depends on factors such as the type of data, audience preferences, and the message being conveyed. Various visualization techniques, including histograms, scatterplots, and dashboards, offer different benefits and limitations depending on the type of data you are presenting.
Limitations of Data Analytics
Despite its numerous benefits, data analytics has inherent limitations that accounting and finance professionals must be aware of.
One glaring limitation involves a lack of good data quality. Data quality issues, such as inaccuracies, inconsistencies, and biases, can affect the reliability of analysis results.
Additionally, data analytics techniques may not always capture the full complexity of real-world phenomena, leading to oversimplification or misinterpretation of findings.
How to Gain Skills in Data Analytics, Today
Data analytics is complex, but when used accurately and efficiently, it provides accounting and finance professionals the opportunity to present actionable insights and make informed decisions in their professional roles.
This article is a top-level overview of the power of data analytics—if you are ready to learn more and dive deeper into data, don’t miss CPE Flow’s Data Analytics course.
This CPE course for accounting and finance professionals launches on April 10th, but if you enroll during our presale, you’ll receive the full course for just $47, a 75% discount.
Do you have questions? I’m happy to answer—just reach out, and we’ll get back to you as quickly as possible.
Thank you for reading,

Nathan Liao, CMA
Empty space, drag to resize
Nathan Liao, a Certified Management Accountant, educator, and influential business figure in the accounting industry, has dedicated over a decade to supporting more than 82,000 accounting and finance professionals in their pursuit of the CMA certification. As the visionary founder of CMA Exam Academy and CPE Flow, Nathan is committed to delivering premier online training solutions for the next generation of accounting and finance professionals.
Explore Our Self-Paced CPE Courses
CPE Flow
© 2022 - 2024 CPE Flow, LLC. All Rights Reserved.
Refund & Complaint Policies
Requests for refunds must be made in writing within 5 days of purchasing the course. No refunds or credits will be provided after this point or if the final assessment is completed. CPE Flow issues refunds within 30 days in the same format as the payment was received. The refund amount is based on the timeframe in which the request was submitted (see table).
Request Timeframe |
Refund/Credit Amount |
≤ 2 Hours |
100% Refund* |
> 2 Hours ≤ 48 Hours |
90% Refund* or 100% Credit** |
> 48 Hours ≤ 5 Days |
0% Refund or 50% Credit** |
* If a credit card was used for purchase, the 3.5% credit card fee will be deducted from the refund amount.
**Credit can be applied towards the same offering at a later date or a different offering.
For complaints or concerns, please email support@cpeflow.com